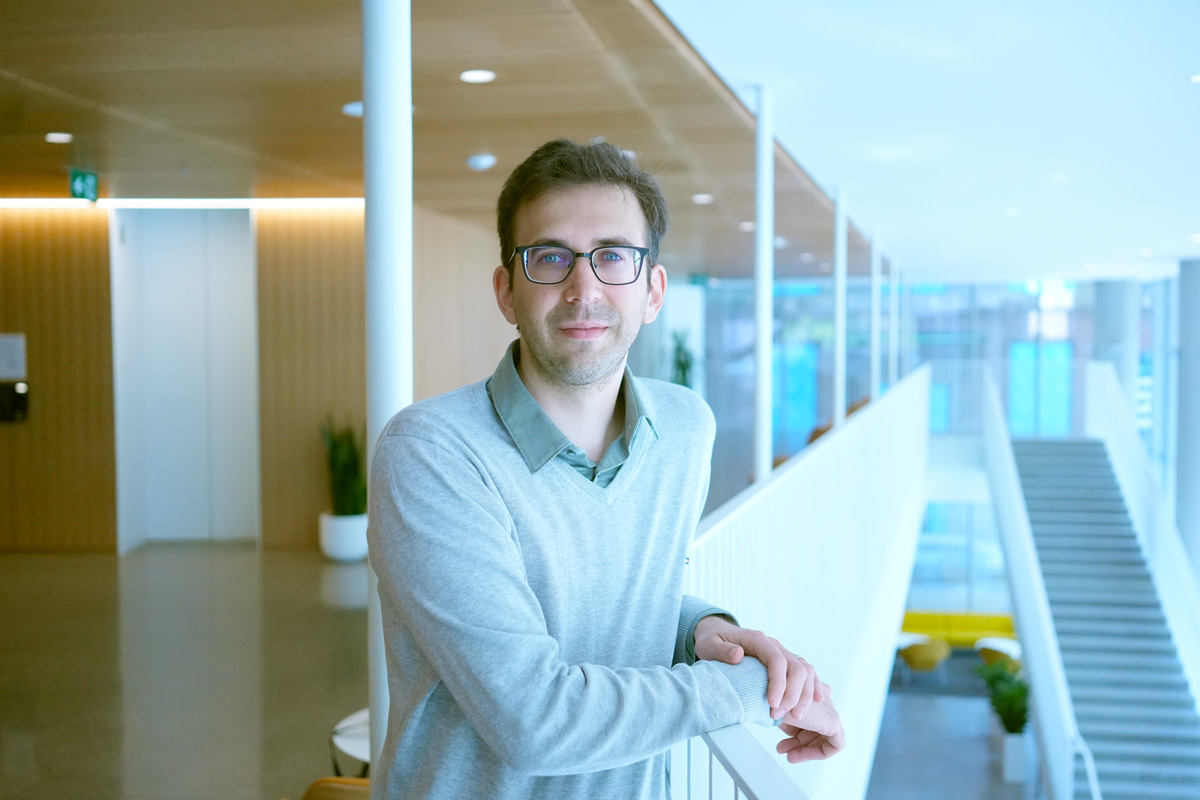
MAY 7, 2024 • By Matthew Tierney
ECE professor Nicolas Papernot’s project on a technical framework for future AI regulation was recently awarded an AI2050 Schmidt Sciences Early Career fellowship.
The project builds on multidisciplinary collaborations between Papernot — who is cross-appointed with the Department of Computer Science, a faculty member at the Vector Institute and a faculty affiliate at the Schwartz Reisman Institute — Professor Lisa Austin from University of Toronto’s Faculty of Law, and Professor Xiao Wang of Northwestern University’s Department of Computer Science.
The team is exploring how a protocol borrowed from cryptography, called zero-knowledge proof (ZKP), can verify whether an AI model was developed in compliance with certain rules. Many governments around the world, including in Canada, are preparing legislation to address the growing power of AI.
Papernot presented preliminary research results to a standing committee at the House of Commons last fall.
“Technology of course can be used for good or for bad. Regulation would not only discourage the negative use cases but also provide incentive for the positive ones,” says Papernot.
On the one hand, threats such as information manipulation — which AI can do at unprecedented scale — may well test the foundations of our modern democracies. On the other, institutions that use AI will want a way to demonstrate good faith with their constituents.
“Increasingly, companies, hospitals and governments will have to give evidence that their AI models are behaving in ways that comply with what the law requires, whether that’s related to privacy, security, and so on,” says Papernot.
The question that drives Papernot and his collaborators is: how can they prove it?
Currently, auditing tools do exist for machine learning (ML) and deep learning (DL) algorithms, the engines of AI. But even assuming there’s mutual trust between the developer and the auditor, information sharing can be hampered by proprietary, privacy or security concerns, either of the algorithms or the data the algorithms were trained on.
A complicating factor is that developers themselves don’t always understand the paths their own AI models take to reach their end results.
The ZKP solution would allow developers to prove that they used certain pieces of data without exposing the data itself.
“So, for example, if someone were to ask, ‘Was my data used in this model?’ the developer can answer confidently without revealing the other data points.”
Cryptographic guarantees are typically resource-intensive to incorporate after the fact. The team has designed their protocols with simple building blocks so they can be implemented alongside the algorithm during development. This will require early buy-in from the developer, and that is not the only potential stumbling block.
“The protocols require a different process than the ones most currently used in AI pipelines,” says Papernot. “For instance, the developer can’t take advantage of GPUs.”
Papernot believes that developers will be motivated to opt-in because the public’s demand for fairness or privacy guarantees from AI models will make them look to other providers if they’re absent.
“Self-interest is a better motivation rather than being forced to do something,” he says.
These certifications could be handled by a global regulatory body, he adds, similar to other domains where coordination is essential across borders, such as the aviation industry,
Another regulatory role model might be the International Organization for Standardization (ISO). This body certifies that participating companies have followed approved systems and processes for their products or services. The companies then use the certification to reassure consumers about the quality and safety of their product.
“As AI technology continues to evolve, it is imperative to simultaneously advance the regulatory and technological frameworks that ensure its safe and ethical use,” says Professor Deepa Kundur, Chair of ECE. “Professor Papernot’s work is pivotal because it not only mitigates risks, but also maximizes the technology’s application potential, making it a cornerstone for future innovations."
“The scope of what we’re trying to achieve is very ambitious,” says Papernot. “We’re asking how AI will impact society, essentially. It’s hard to do that from just one discipline’s perspective. Thankfully, the Schimdt Sciences organization has stepped up to provide the space needed for such highly exploratory and complex research.”